RETHINKING EVALUATION: USING BIG DATA AND OTHER INNOVATIVE TOOLS AMIDST CRISES
- January 12, 2024
- Posted by: sarangmangi
- Category: Insights
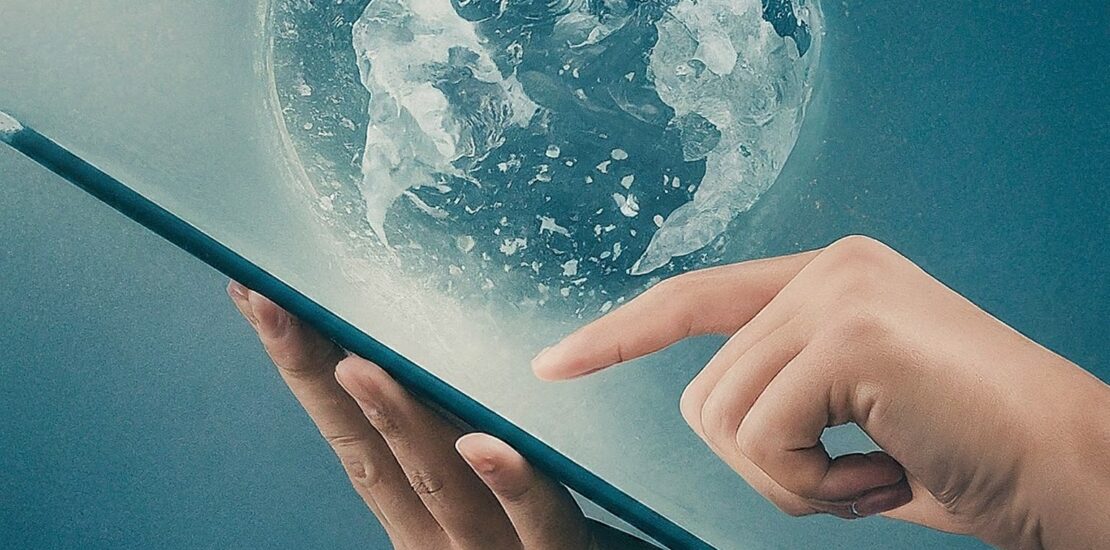
(This post is based on a guidance note prepared for UNODC. Here is the link to the original full report.)
Most evaluations carried out by UN utilize primary data collection involving field missions, direct observations, surveys and interviews with the key informants. While these methods provide invaluable information to the evaluation teams, IES now has a major opportunity to expand its toolbox beyond these perceptual methodologies.
While UN has a number of options that can be employed for evaluation during crisis, this note discusses nine conventional and non-conventional options, which are organized by level of complexity from the most basic to the most complex options available to the IES. These options include Self-assessment, Content analysis, Online tools, Radio surveys, National services, Archival data, Crowdsourcing, Experiments and Big data evaluations. Table 1 below presents a summary SWOT analysis, which is followed by brief discussion.
Self-assessments by field offices can be used to supplement, and in extreme cases, even replace, other data sources and methods. It assumes that field offices retain some ability to monitor and compile self-assessment reports despite limitations pertaining to travel and safety. In addition to being cheap and safe, this option is very good at utilizing monitoring data and staff inside knowledge and insights. It’s best used when no other option is available for an intermediate solution or only to provide an additional datapoint for triangulating findings. Surprisingly, formal content analysis of documents (e.g., policy recommendations included in actual legislations) is rarely used in UNODC’s evaluation work. It can not only offset some of the limitations associated with the inability to travel and directly interact with and observe stakeholders in action, but also provide in-depth information on outcomes and impacts. As most public documents are openly available for free, evaluators can go back in time and reconstruct baselines as well as record documentary evidence on changes that have taken place since then. This data also does not suffer from recall bias as it is recorded on an ongoing basis, however the possibility of biased (i.e., favorable) document selection cannot be ruled out. As commonly used online tools are adequately understood, the focus of the note was on more advanced tools such as Computer-assisted telephone interviewing (CATI) and SurveyCTO. While normal tools are adequate for IES at the moment, more advanced tools could be reconsidered if and when IES becomes involved in a lot of field data collection remotely or respondent safety becomes a concern. While radio is often viewed as a one-way communication platform, some development programmes have used it as a source for complementing feedback from beneficiaries. This methodology is best used for evaluations of project that target populations for whom radio is still main source of information or in hazardous situations (e.g., conflict zones).
Using National services such as local data collectors, evaluators and mobile firms enable IES in creation of local evaluation capacity and reaching non-traditional respondents. These service providers are generally more aware of local context and can provide input to ensure data collection tools and processes are contextualized to the particular population; including language and customs. In addition to being less expensive and safe, the possibility of longitudinal data collection is one of major advantages of using this option. Using local data collectors and mobile firms is a bit easier when the project area and beneficiaries are well-defined. Since countries may have limited numbers of well-known or experienced evaluators, it also risks overburdening local systems and crowding out local governments and users, who may normally pay lesser fees. When using this option, clear protocols on data methodology and collection should be established and agreed with the data collection firms to ensure its quality and reliability as well as for maintaining and respecting the privacy and safety of respondents.
A wide variety of archival data such as crime statistics, census and household surveys, and institutional data are available even in remote parts of the world. UNODC projects and programmes often utilize some of these in the project conception and design phase. However, these data are frequently underutilized, if used at all, for monitoring and evaluation (M&E) purposes. As no travel is needed to acquire these databases, these are safe to collect and use. By going beyond perceptions of a limited number of stakeholders, these datasets can also help IES derive more objective information on the results of programme interventions. These dtaa also enable running advanced analytics, which in turn, can help show programme contribution to results achieved after controlling for all other relevant factors. However, as these data are often collected with other purposes in mind, these may not be optimized for use in evaluations, which can also make showing programme contribution harder. IES can also employ crowdsourcing to collect data, typically pictures and videos on project sites posted by project beneficiaries and local residents.
Experimental designs, and Randomized controlled trials (RCTs) in particular, are considered the gold standard in evaluating the impact of development interventions. As RCTs are very effective for uncovering causality in complex interventions, these are the most appropriate option for conducting impact evaluations. While UNODC’s portfolio of a large number of small projects makes it challenging for IES to undertake many experiments, it can and should be undertaken at the thematic level by combining several related projects into a portfolio (i.e., extremist violence). After years of working in this field, it is critical for UNODC to demonstrate the impact of its work in areas where it has made substantial investments. While RCTs are easier in person, the experience shows that these can also be undertaken remotely.
The recent revolution in artificial intelligence/ machine learning (AI/ML) and blockchain technologies have made it imperative for UNODC/IES to seriously consider using big data. It is a treasure trove of passively generated data from digital devices such as mobile phones, sensors, web searches, Internet banking, news, social media interactions, and satellite imagery. Big data can help evaluators overcome the challenge of ruling out counterfactual scenarios and establish the causal effect of programme interventions. Unlike traditional interviews and surveys that are typically conducted at one point in time, big data is passively collected constantly over long periods of time. This makes it easier to observe longitudinal and nonlinear effects on beneficiary populations. It also allows combining several datasets from widely divergent sources to reveal deeper relationships as well as interactive effects among various interventions. It can also help collect information on sensitive subjects such as household dynamics, domestic violence and sexual preferences, which is something rather difficult to do with traditional methods. Moreover, traditional evaluation methods, including Randomized Control Trials, fail to capture unintended outcomes as they are primarily designed to test whether intended outcomes have been achieved. Big data, on the other hand, can not just enable the project managers to not just detect unintended consequences, but also address them as they occur during the implementation itself.
However, big data also has its own weaknesses and challenges that need to be addressed. First, in the context of international development, using big data necessitates building a new ecosystem, often from the ground up. Firstly, accessing this data requires establishing partnerships with non-traditional service-providers (e.g., satellite images and cell phone records). Second, IES will need to engage data scientists and programmers, who can help process and manage vast volumes of data. Further, use and interpretation of data depends on analytical techniques that are cross-disciplinary in nature. This, in turn, necessitates changes in the role of evaluation managers and evaluators as typical M&E systems are geared only to handling small data such as surveys, interviews and focus groups. Second, algorithms being used with big data are often opaque, unregulated, and biased. Third, despite its large size, big data can be unrepresentative of the underlying population. In many developing countries, even when data such as phone records and ATM transactions are available, it may be less representative as very few people use such services. Extreme poverty and lack of mobile phones, for example, are more likely to correlate with each other. Fourth, given the intimate nature of big data, sufficient resources need to be allocated for data security and anonymization.
Case studies
The standard methodologies used by the IES may not always be feasible, optimal or even necessary. As an example, a thematic evaluation of UNODC’s research programme can almost completely be driven by documentary evidence supplemented with remote surveys and interviews. On the other hand, eLearning and capacity development work requires an experimental approach to truly understand if it is making any difference. Other evaluations such as on money-laundering and wildlife crime would benefit from big data evaluations using data from, among others, financial and cellphone records, social media sentiment analysis and remote sensing applications. However, to do so, IES will need to both strengthen their own capacity to use more advanced options such as RCTs and big data as well as engage in extensive partnerships within and beyond the UN system. These case studies are discussed at some length in the guidance note.
CONCLUSION
Given the challenging conditions under which IES is currently operating, IES needs to explore both conventional and non-conventional options for conducting evaluations. Several of these options do not require much additional capacity development for the IES, however conducting experiments and using big data evaluations will require building a whole new ecosystem including partnerships with academia and private sector organizations. IES will also need to involve various UNODC programmes and projects more closely in developing their capacity for monitoring and evaluation, especially where they are expected to contribute ‘self-assessments’ or provide information to develop theories of change for the RCTs. As use of big data is more cost effective when used for both real-time monitoring and evaluation, this will be of paramount importance. In this context, it is important to note that the programme of work adopted by the United Nations System Chief Executives Board for Coordination (CEB) in 2015 adopted a comprehensive United Nations system approach to the data revolution. It encourages all UN entities to ensure that timely, accurate and reliable data informs policymaking. However, big data can supplement, but not substitute (with some exceptions) for existing best practices on evaluation. It can play a vital role in evaluation if evaluators take great care in asking the right questions on generation, processing, analysis and use of data. It requires focusing on quality as much as on quantity of data.
IES could initiate the process of integrating big data with an experimental pilot study. Given the complex interlinkages involved in money-laundering or drugs dependence treatment, these evaluations may be the right candidates for piloting big data evaluations. Similarly, an evaluation of UNODC’s work in thematic areas where UNODC had made substantial investments (e.g., eLearning) could be used for piloting an experimental design evaluation.